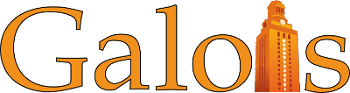
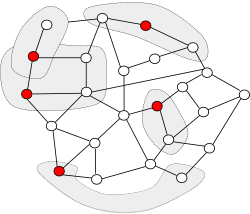
What is Galois?
The Galois system permits application programmers to exploit amorphous data-parallelism in irregular algorithms without having to write explicitly parallel code. Irregular algorithms operate on complex data structures like graphs, priority queues, and lists, and they are the norm in important problem domains like machine learning, graph analytics, electronic design automation (EDA), and network security.
The Galois programming model provides a small number of programming patterns that are implemented in C++. The Galois library provides concurrent data structures, schedulers, and memory allocators. The Galois runtime executes these programs in parallel, using parallelization strategies such as optimistic and round-based execution. Galois runs on shared-memory NUMA platforms and NVIDIA GPUs. A subset of the Galois programming model is supported on distributed-memory machines.
What is Galois used for?
- Graph analytics
- Lonestar and LonestarGPU have programs for the usual problems. Galois
does particularly well on high-diameter graphs like road networks, circuits,
and provenance graphs because it supports richer scheduling policies than
other systems in this space.
-
D-Galois is a state-of-the-art distributed graph analytics system built
on top of Galois that leverages temporal and structural invariants of graph
partitioning to optimize communication. It can be used to write
efficient distributed algorithms such as a
provably round-efficient implementation of betweenness-centrality.
- Galois was a HPEC Graph Challenge 2017 Champion and 2019 Student Innovation Award Winner.
- Lonestar and LonestarGPU have programs for the usual problems. Galois
does particularly well on high-diameter graphs like road networks, circuits,
and provenance graphs because it supports richer scheduling policies than
other systems in this space.
- Electronic Design Automation (EDA)
- Logic synthesis.
- Maze routing.
- Global routing of circuits.
- Asynchronous circuit design tools: DARPA IDEA/POSH project led by Yale University.
- Logic synthesis.
- Graph mining
- Galois can be used for graph pattern mining (GPM) applications such as motif
counting, frequent subgraph mining, and k-clique listing.
Pangolin
is an efficient graph pattern mining framework built on top
of Galois that provides high level abstractions for users to write
GPM applications without compromising performance.
- Galois can be used for graph pattern mining (GPM) applications such as motif
counting, frequent subgraph mining, and k-clique listing.
Pangolin
is an efficient graph pattern mining framework built on top
of Galois that provides high level abstractions for users to write
GPM applications without compromising performance.
- Scientific computing
- Guaranteed quality 2-D mesh generation and refinement: Lonestar benchmarks.
- Metis graph partitioner: Lonestar benchmark.
- Iso-geometric finite elements.
- Benchmarking studies
- Studies at HP Enterprise: ICPE 2016, MASCOTS 2016.
- Studies on supercomputing clusters Stampede2 and Bridges: VLDB 2018, IPDPS 2020
- Real-time intrusion detection in computer networks
- Engine for Industrial Graph System
- The Katana Graph engine uses Galois as its graph processing backend; Katana Graph combines Galois with state-of-the art storage and hardware technologies to provide efficient, scalable, industry grade graph processing to its customers.
How do I learn more about Galois?
- Concepts
- The TAO of parallelism in algorithms: PLDI 2011
- Optimistic parallelism requires abstractions: PLDI 2007
- Galois on shared-memory NUMA platforms
- A lightweight infrastructure for graph analytics: SOSP 2013
- Scaling runtimes for irregular algorithms to large-scale NUMA systems: IEEE Computer 2015
- Galois on Intel Optane DC Persistent Memory
- Galois on NVIDIA GPUs
- Galois on distributed-memory platforms
Download
The latest release along with instructions on how to install the Galois system and run a sample benchmark application can be found here.
Contact
For questions or comments about the Galois system, please contact galois-users@utlists.utexas.edu.